Evidence@AI: Embedding Digital Evidence Autonomously for Legal Foundations in the AI Era
- Larry Chou
- Mar 26
- 3 min read
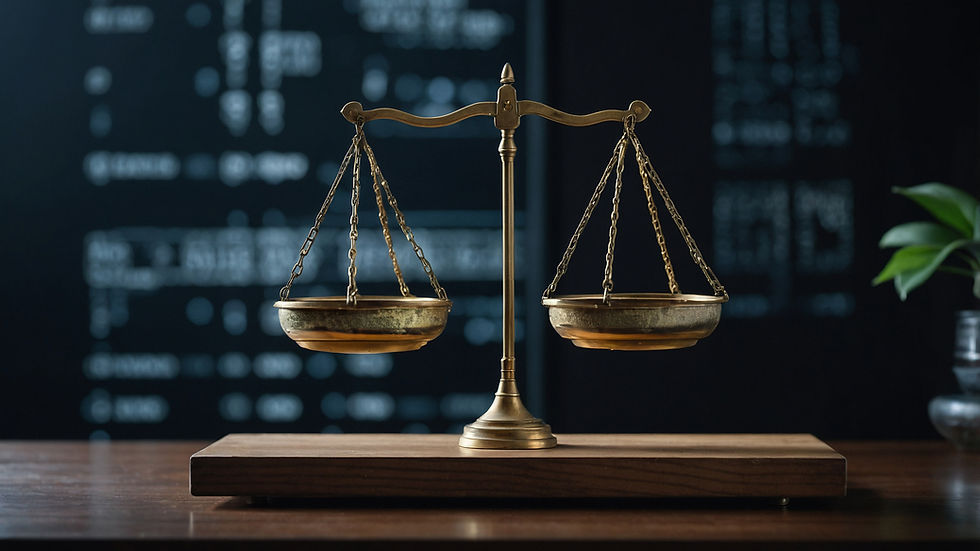
Abstract
Integrating artificial intelligence (AI) into critical sectors demands re-evaluating evidentiary standards. This paper introduces "Evidence@AI," a framework to establish robust legal foundations for AI-generated evidence. This framework ensures traceability, verifiability, and third-party certification by leveraging the concept of "digital fingerprints" embedded autonomously within AI outputs, coupled with hardware root of trust (HRoT). We argue for a layered approach, emphasizing immutable provenance logs and embedded digital fingerprints, to secure the reliability and admissibility of AI evidence in legal proceedings.
1. Introduction
Professor Rothstein, a rigorous evidentiary framework becomes paramount as we navigate the complexities of AI's increasing autonomy. Traditional legal principles, while foundational, require adaptation to address the unique challenges posed by AI's "black box" nature. This paper proposes "Evidence@AI," a system that embeds digital evidence autonomously, creating a bridge between established legal doctrines and the realities of the AI era.
2. The Evidentiary Challenge of AI's Opacity:
The opacity of many AI systems and intense learning models raises significant concerns regarding bias, manipulation, and error. To address this, "Evidence@AI" employs "digital fingerprints" to trace the origin and provenance of AI outputs, ensuring transparency and accountability.
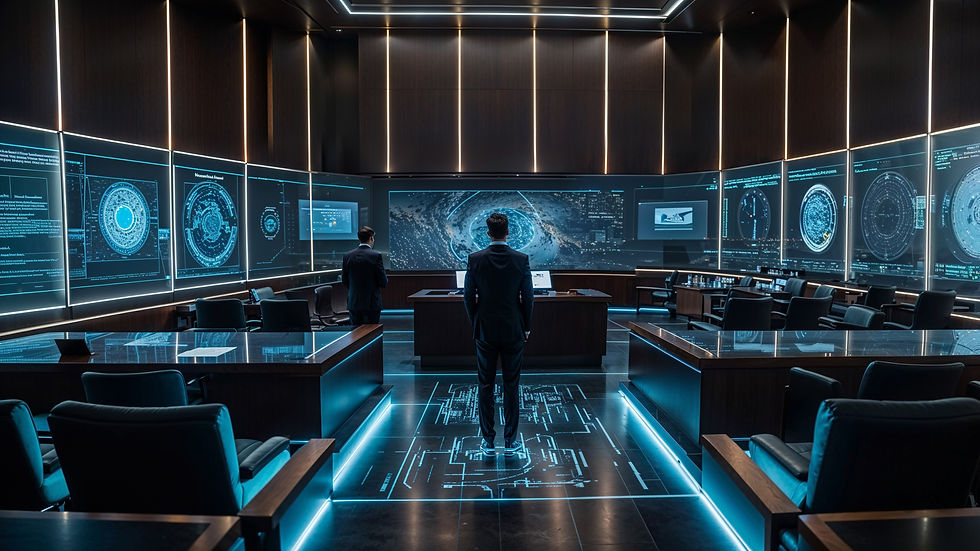
3. Evidence@AI: Digital Fingerprints, Hardware Root of Trust, and Autonomous Embedding:
Digital Fingerprints:
"Evidence@AI" generates unique cryptographic identifiers ("digital fingerprints") for all interactions within an AI system, including data inputs, algorithms used, and human interactions.
These fingerprints, generated using cryptographic hashes and digital signatures, ensure immutability and authenticity.
They form a verifiable chain of custody, from data input to AI output.
Hardware Root of Trust (HRoT):
An HRoT provides a secure, hardware-based foundation for establishing the identity and integrity of the AI system.
It ensures secure boot, integrity measurement, and key management, which are critical for cryptographic operations.
The HRoT provides the initial digital fingerprint for the hardware and the data it processes.
Autonomous Embedding:
"Evidence@AI" autonomously embeds relevant digital fingerprints directly into the AI output.
This enables independent verification of provenance and integrity, using techniques like digital watermarking and secure metadata.
This embedding allows for direct examination of the origin of the data.
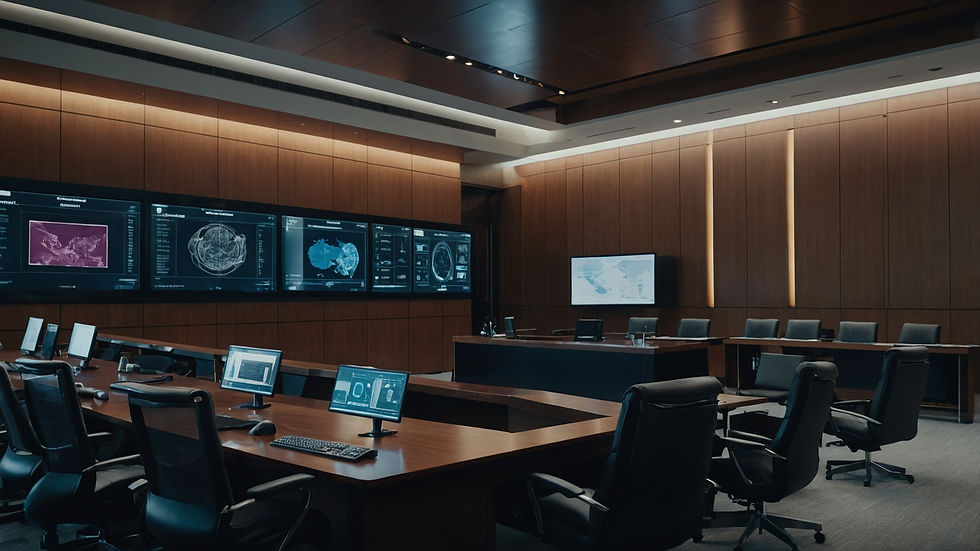
4. Evidentiary Principles of Evidence@AI:
To ensure the reliability of AI evidence, "Evidence@AI" adheres to the following principles:
Traceability through Digital Fingerprints:
Detailed provenance logs, using digital fingerprints, trace all interactions and data transformations.
Verifiability:
Independent verification through robust testing, embedded fingerprints, and formal verification.
Third-Party Certification:
Independent certification of AI systems and provenance logs, potentially using blockchain technologies.
Relevance and Reliability:
Adherence to traditional evidentiary standards of relevance and reliability, supported by expert testimony.
Legal Admissibility:
Development of new legal frameworks to address the admissibility of AI evidence.
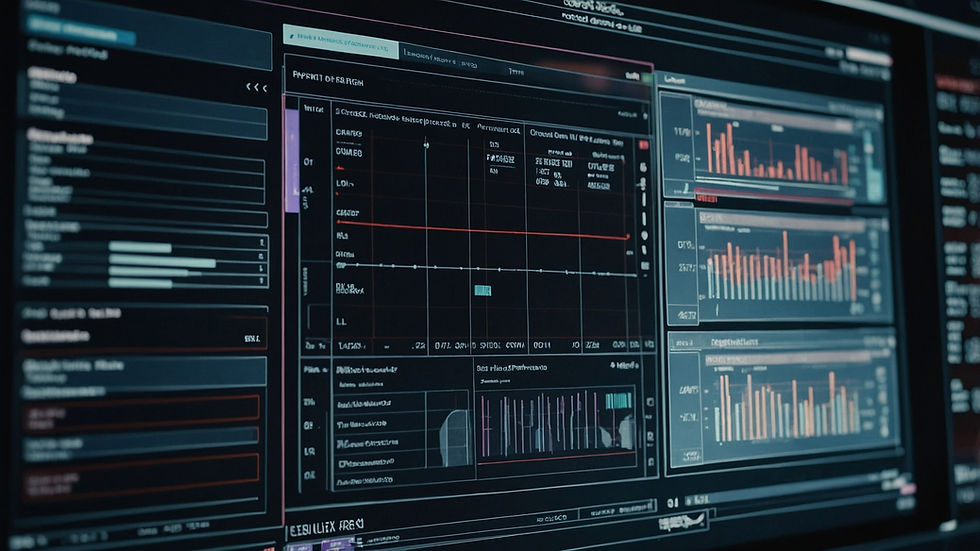
5. A Layered Framework for Evidence@AI:
"Evidence@AI" employs a layered approach:
Layer 1: System Integrity (HRoT and Digital Fingerprinting):
Secure design and development, incorporating HRoT and digital fingerprinting.
Layer 2: Output Validation (Provenance Logs and Embedded Fingerprints):
Verify output accuracy and log integrity using embedded fingerprints.
Layer 3: Contextual Relevance:
Expert testimony to establish the relevance of AI output within the legal context.
6. Legal and Ethical Considerations:
Due process, privacy, bias mitigation, and accountability are paramount.

7. Conclusion:
"Evidence@AI" provides a crucial foundation for applying traditional legal frameworks in AI. By autonomously embedding digital evidence, we ensure traceability, verifiability, and trustworthiness, fostering legal certainty in a technologically advanced landscape. This framework will be of significant interest to you, Professor Rothstein, as we collectively navigate the evolving legal landscape of AI.