Safety@AI
- Larry Chou
- Mar 26
- 9 min read
Ensuring AI Safety and Accountability Through Traceability and Verification
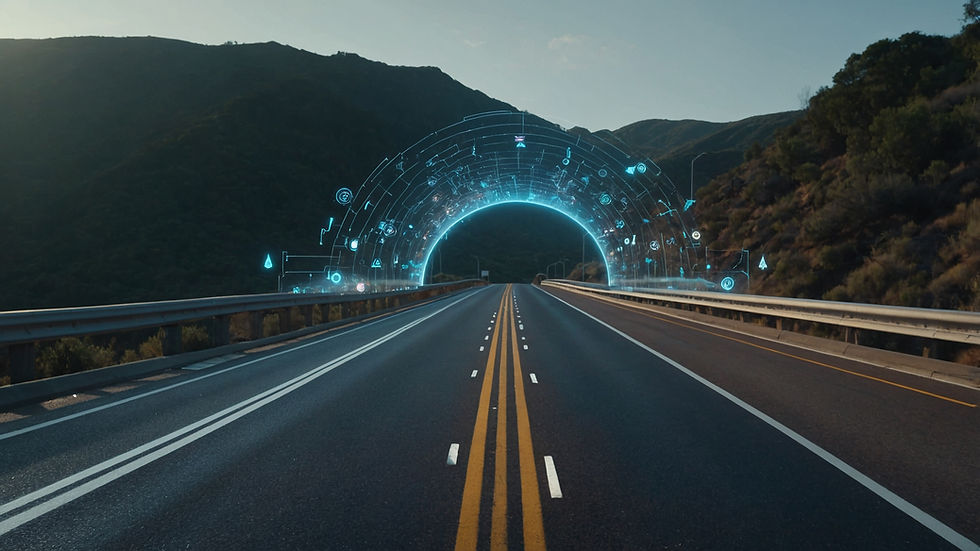
Learning from Other Industries
Introduction
Artificial intelligence (AI) is rapidly transforming various aspects of our lives, from healthcare and finance to transportation and entertainment. As AI systems become more sophisticated and integrated into critical applications, ensuring their safety and accountability becomes paramount. This report explores the concept of AI traceability and verification, drawing parallels from established practices in industries like food, pharmaceuticals, and manufacturing to argue that tracing and verifying AI's origin, authenticity, and integrity are crucial steps toward building safe and accountable AI systems.
Safety and Accountability Challenges in Traditional Industries
Before delving into the specifics of AI, it's essential to understand how traditional industries have addressed safety and accountability challenges. Examining their experiences can provide valuable insights for the development and deployment of AI systems. The challenges faced by traditional industries in ensuring safety and accountability offer valuable lessons for the development and deployment of AI systems.
Food Industry
The food industry faces numerous safety challenges, primarily related to contamination and spoilage. Contamination can occur at any stage of the food production process, introducing harmful bacteria, viruses, or toxins that can cause illness 1. Food spoilage, often caused by improper handling or storage, can render food unappetizing and potentially dangerous1. To mitigate these risks, the food industry has implemented stringent safety measures, including proper handwashing, sanitation, and temperature control2.
Accountability in the food industry is crucial for maintaining consumer trust and preventing foodborne illnesses. Companies are held responsible for the safety and quality of their products, and failures can lead to recalls, legal action, and reputational damage3. Issues such as misleading labeling, unsustainable practices, and lack of transparency can also raise concerns about accountability in the food industry.
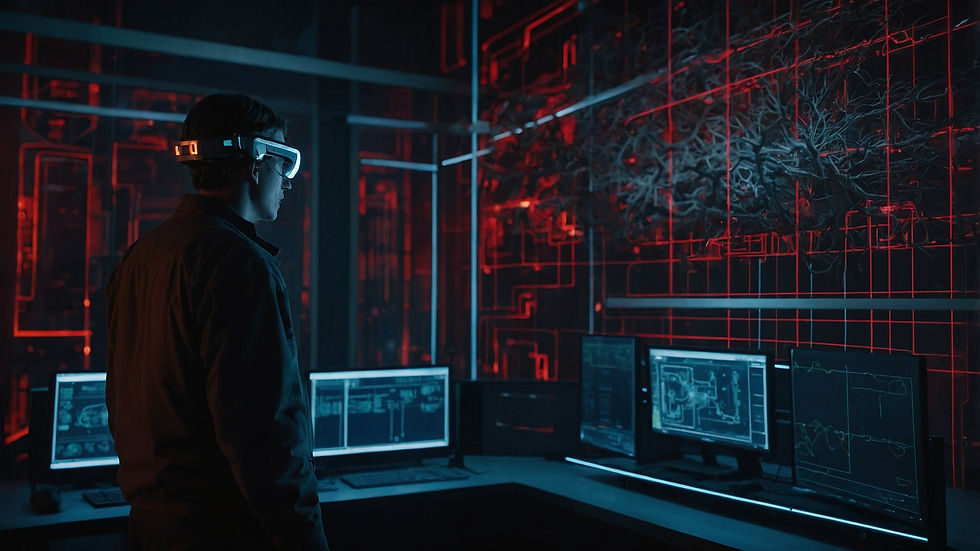
Pharmaceutical Industry
The pharmaceutical industry deals with products that directly impact human health, making safety and accountability even more critical. Safety issues in this industry can arise from various factors, including incorrect dosage, allergies to ingredients, drug interactions, and improper storage6. Manufacturing processes must adhere to strict quality control standards to prevent contamination and ensure product integrity7.
Accountability in the pharmaceutical industry extends beyond product safety to encompass ethical considerations, access to medicines, and transparency in research and development8. Companies are expected to conduct rigorous clinical trials, accurately report findings, and ensure that their products are accessible and affordable to those who need them9.
Manufacturing Industry
The manufacturing industry faces a wide range of safety hazards, including those related to machinery, chemicals, electricity, and ergonomics10. Accidents, injuries, and even fatalities can occur due to improper machine guarding, inadequate safety training, or failure to follow safety procedures12.
Accountability in manufacturing involves ensuring product quality, worker safety, and environmental responsibility. Companies are expected to comply with safety regulations, maintain accurate records, and implement measures to prevent workplace accidents and environmental damage13.
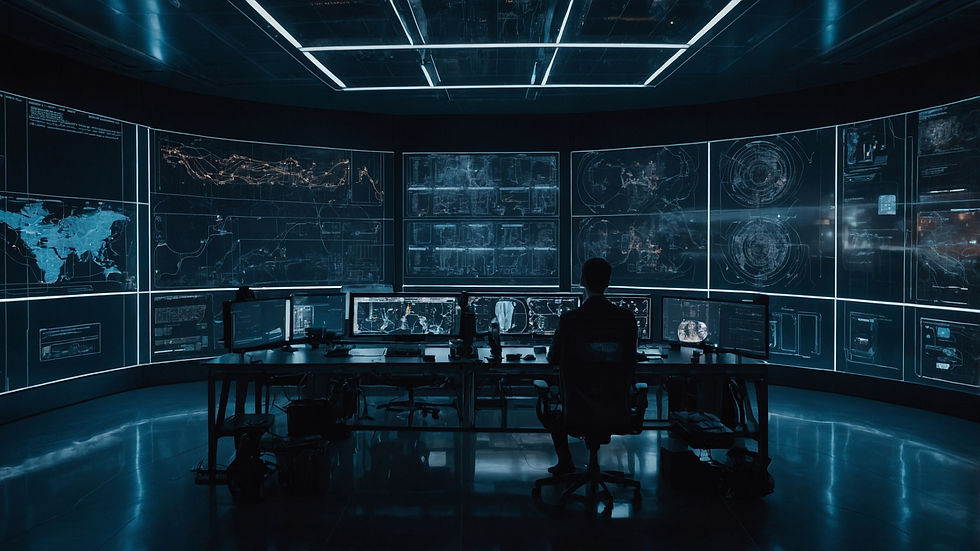
Traceability and Verification in Traditional Industries
To address safety and accountability concerns, traditional industries have implemented various traceability and verification mechanisms. These mechanisms provide a means to track products throughout the supply chain, verify their authenticity, and ensure their integrity.
A common method used for traceability in these industries involves unique identification of products or batches. This can be achieved through various technologies, including barcodes, RFID tags, and direct part marking. These identifiers are attached to each product or batch, allowing for tracking throughout the supply chain. Each time a product or batch is scanned or its identifier is read, data is transmitted to a central database, providing a comprehensive record of its journey. This enables companies to quickly identify the origin of a product, trace its movement, and take necessary actions in case of recalls or safety concerns.
Food Industry
In the food industry, traceability methods like barcode scanning, RFID tags, and DNA analysis are used to track products from farm to table 14. This helps identify potential sources of contamination and facilitate efficient recalls if necessary16. To verify the authenticity and integrity of food products, techniques such as DNA testing, spectroscopy, and chromatography are employed to identify adulteration, mislabeling, and ensure that products meet quality standards17.
Pharmaceutical Industry
Pharmaceutical companies utilize serialization, assigning unique identifiers to individual product units, to track and trace medications throughout the supply chain19. This helps prevent counterfeiting, ensure product authenticity, and facilitate efficient recalls21. For example, the European Union's Falsified Medicines Directive (FMD) utilizes a system for verifying the authenticity of medications using 2D barcodes in line with the specifications of GS122.
Pharmacies and authorized bodies scan the barcode and verify it via the European Medicines Verification System (EMVS) before dispensing the medication. To further ensure the integrity of pharmaceuticals, companies use tamper-evident packaging, which provides visual evidence if a product has been opened or altered23. Other methods for verifying authenticity include holograms and digital systems that integrate with databases of authentic medications24.
Manufacturing Industry
Manufacturers use various traceability methods, including barcodes, RFID tags, and direct part marking, to track components and finished products throughout the production process26. This helps ensure product quality, identify potential defects, and improve supply chain efficiency28. To verify product authenticity and integrity, manufacturers employ techniques such as visual inspection, quality control checks, and advanced testing methods like X-ray inspection and ultrasonic testing29.
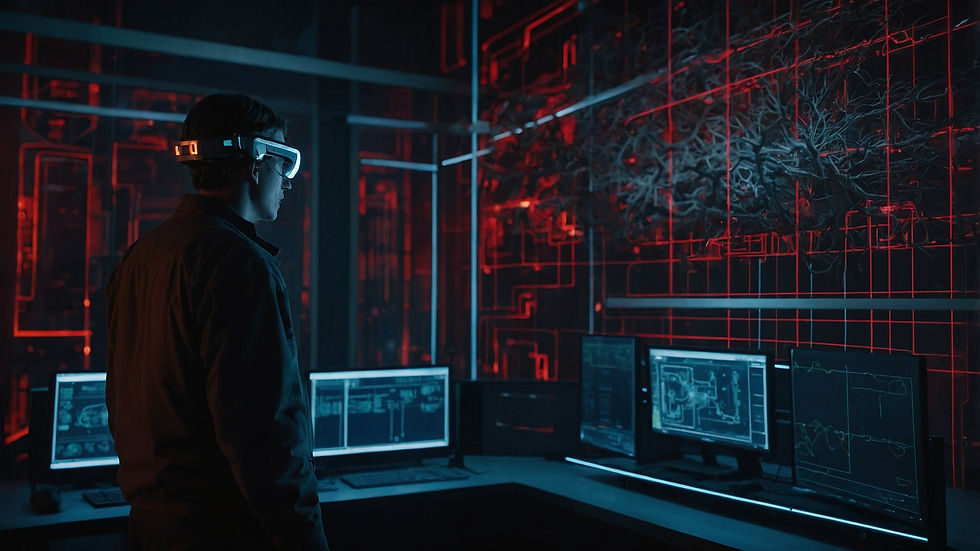
Parallels Between Traditional Industries and AI
Just as contaminated food or a faulty drug can have serious consequences, AI systems that malfunction or exhibit unintended behavior can pose significant risks30. The safety and accountability challenges faced by traditional industries share similarities with those emerging in the field of AI. The lessons learned from these industries, particularly in implementing traceability and verification mechanisms, can be applied to AI development to mitigate potential risks and ensure responsible use.
AI Safety and Accountability Challenges
AI systems can be vulnerable to various issues, including:
Bias and Discrimination: AI models trained on biased data can perpetuate and amplify existing societal biases, leading to unfair or discriminatory outcomes. For example, an AI system used for loan applications might unfairly deny loans to individuals from certain demographic groups if the training data reflects historical biases in lending practices.
Lack of Transparency: The complexity of some AI algorithms can make it difficult to understand how they arrive at their decisions, hindering accountability and trust. This "black box" nature of AI can make it challenging to identify and correct errors or biases, potentially leading to harmful consequences.
Unintended Consequences: AI systems may exhibit unexpected or unintended behaviors, especially when deployed in complex real-world environments. For instance, an AI system designed to optimize traffic flow might inadvertently create unexpected congestion patterns or prioritize certain routes over others, leading to unintended disruptions.
Security Risks: AI systems can be vulnerable to cyberattacks, data breaches, and manipulation, potentially leading to harmful consequences. Hackers could exploit vulnerabilities in AI systems to gain access to sensitive data, alter AI outputs, or disrupt critical infrastructure controlled by AI.
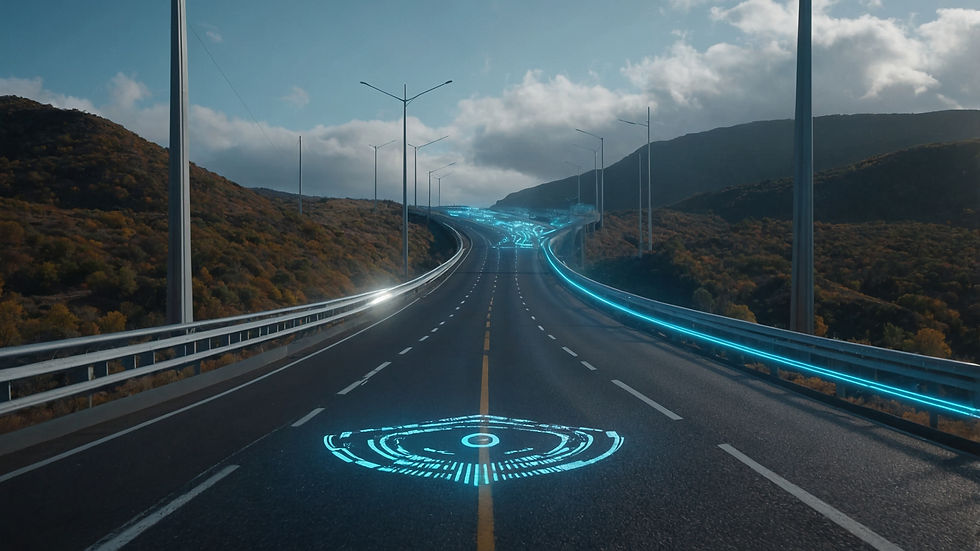
Applying Traceability and Verification to AI
To address these challenges, the principles of traceability and verification can be applied to AI systems. This involves:
Tracing the Origin of AI Models: Documenting the data sources, algorithms, and development processes used to create AI models. This includes maintaining a record of the training data, the AI model's architecture, and the various stages of its development and deployment.
Verifying AI Authenticity: Ensuring that AI models are genuine and have not been tampered with or corrupted. This can involve using digital signatures, cryptographic techniques, or other methods to verify the integrity of AI models and prevent unauthorized modifications.
Maintaining AI Integrity: Ensuring that AI models continue to function as intended and do not deviate from their original design or purpose. This requires ongoing monitoring and evaluation of AI systems to detect any drift in performance or unexpected behaviors.
Furthermore, applying traceability and verification to AI can help address the issue of explainability. Understanding how an AI model arrives at its decisions is crucial for holding it accountable31. By tracing the decision-making process of an AI system, we can gain insights into its reasoning and identify potential biases or errors.
Existing Frameworks and Initiatives for AI Traceability and Verification
Several frameworks and initiatives are emerging to address AI traceability and verification. These include:
NIST AI Risk Management Framework (AI RMF): Provides guidance for managing risks associated with AI, including those related to transparency and accountability33.
ACT-IAC Ethical Application of AI Framework: Offers a framework for the ethical development and implementation of AI, including considerations for data accountability and algorithm explainability34.
W3C PROV: A general purpose recommendation for provenance that provides a foundation for maintaining a complete account of the origin and history of data, processes, and artifacts involved in the production of an AI model35.
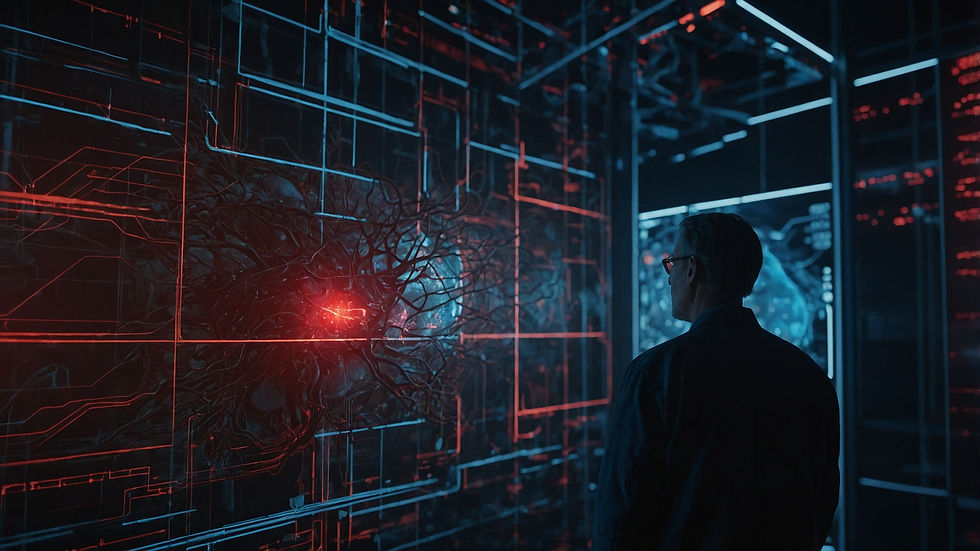
Potential Benefits and Limitations of AI Traceability and Verification
Implementing AI traceability and verification frameworks can offer several benefits:
Enhanced Trust and Accountability: Traceability and verification can increase trust in AI systems by providing transparency and ensuring they are developed and used responsibly.
Improved Safety: By tracking the origin and development of AI models, potential biases and vulnerabilities can be identified and addressed, leading to safer AI systems. Understanding the origin and development process of an AI model makes it possible to anticipate and mitigate potential unintended consequences.
Facilitated Auditing and Compliance: Traceability and verification can facilitate audits and ensure compliance with regulations and ethical guidelines. Data lineage, which traces data from its origin to its final use in the AI model, can be particularly helpful in ensuring regulatory compliance36.
However, there are also limitations to consider:
Complexity and Cost: Implementing comprehensive traceability and verification mechanisms can be complex and costly, especially for organizations with limited resources.
Potential for Over-Reliance: Over-reliance on traceability and verification may create a false sense of security and neglect other essential aspects of AI safety and accountability.
Conclusion
As AI becomes increasingly integrated into our lives, ensuring its safety and accountability is crucial. By learning from the experiences of traditional industries and applying the principles of traceability and verification, we can take significant steps toward building trustworthy and responsible AI systems. While challenges remain, the potential benefits of AI traceability and verification are substantial, paving the way for a future where AI technologies are developed and deployed in a manner that benefits humanity while minimizing risks. Further research is needed to develop standardized methodologies and best practices for AI traceability and verification across different domains and applications.
Works cited
1. Food Safety Challenges - TSC Associates, accessed January 7, 2025, https://www.tscassociates.com/food-safety-challenges/
2. Top 10 Food Safety Issues - The Association of Nutrition & Foodservice Professionals, accessed January 7, 2025, https://www.anfponline.org/news-resources/top-10-resource-lists/top-10-food-safety-issues
3. Recalls and Outbreaks | FoodSafety.gov, accessed January 7, 2025, https://www.foodsafety.gov/recalls-and-outbreaks
4. Lack of industry regulation and accountability pushing people towards unhealthy eating, accessed January 7, 2025, https://foodfoundation.org.uk/press-release/lack-industry-regulation-and-accountability-pushing-people-towards-unhealthy-eating
5. Consumer Rights & Corporate Accountability - FarmSTAND, accessed January 7, 2025, https://farmstand.org/consumer-rights-corporate-accountability/
6. Medicines - safety issues - Better Health Channel, accessed January 7, 2025, https://www.betterhealth.vic.gov.au/health/conditionsandtreatments/medicines-safety-issues
7. 5 Top Safety Risks in Pharmaceutical Manufacturing - International Enviroguard, accessed January 7, 2025, https://int-enviroguard.com/blog/5-top-safety-risks-in-pharmaceutical-manufacturing/
8. Issues | The Pharmaceutical Accountability Foundation, accessed January 7, 2025, https://www.pharmaceuticalaccountability.org/issues/
9. Increasing transparency and accountability in national pharmaceutical systems - PMC, accessed January 7, 2025, https://pmc.ncbi.nlm.nih.gov/articles/PMC6239016/
10. safetyculture.com, accessed January 7, 2025, https://safetyculture.com/topics/manufacturing-safety/manufacturing-hazards/#:~:text=Chemical%20Hazards,and%20other%20serious%20health%20issues.
11. Top 5 Safety Hazards In the Manufacturing Industry - Evotix, accessed January 7, 2025, https://www.evotix.com/resources/blog/top-5-safety-hazards-in-the-manufacturing-industry
12. Top 10 Safety Violations in Manufacturing, accessed January 7, 2025, https://safetyplus.io/top-10-safety-violations-in-manufacturing/
13. How to Achieve Visibility, Traceability and Accountability in Manufacturing - Parsable, accessed January 7, 2025, https://parsable.com/blog/quality/how-to-achieve-visibility-traceability-and-accountability-in-manufacturing/
14. Food Traceability: What is it + Why It's Important - Inecta, accessed January 7, 2025, https://www.inecta.com/blog/food-traceability-what-is-it-why-its-important
15. Top 5 food traceability methods you need to know - FoodChain Magazine, accessed January 7, 2025, https://foodchainmagazine.com/news/top-5-food-traceability-you-need-to-know/
16. Food Traceability: A Comprehensive Guide, accessed January 7, 2025, https://foodready.ai/blog/food-traceability/
17. Food Authenticity | What is it & Why is it Important? - High Speed Training, accessed January 7, 2025, https://www.highspeedtraining.co.uk/hub/what-is-food-authenticity/
18. Food authenticity testing: A positive approach in combating food fraud - IFIS, accessed January 7, 2025, https://www.ifis.org/blog/food-authenticity-testing
19. The Importance Of Track & Trace In Pharma Manufacturing | Tulip, accessed January 7, 2025, https://tulip.co/blog/track-trace-in-pharma-manufacturing/
20. Types of Traceability in Pharmaceutical Industry - SS Datacode, accessed January 7, 2025, https://datacode.co.in/types-of-traceability-in-pharmaceutical-industry/
21. Why Pharma Traceability is Critical in Healthcare Packaging, accessed January 7, 2025, https://www.healthcarepackaging.com/logistics/traceability-serialization/article/22868047/why-pharma-traceability-is-critical-in-healthcare-packaging
22. Traceability of medicinal products - Uhlmann, accessed January 7, 2025, https://www.uhlmann.de/en/insights/highlights/traceability
23. Confirmed: Key strategies for maintaining integrity in pharma supply chains - theafricalogistics.com, accessed January 7, 2025, https://theafricalogistics.com/confirmed-key-strategies-for-maintaining-integrity-in-pharma-supply-chains/
24. How Pharmacies Ensure Medication Authenticity - medi, accessed January 7, 2025, https://medi51.mypixieset.com/blog/how-pharmacies-ensure-medication-authenticity/
25. Enhancing Pharmaceutical Product Security - Veritech, accessed January 7, 2025, https://veritech.in/blog/enhancing-pharmaceutical-product-security/
26. Traceability in Manufacturing: The Expert's Guide - Metalphoto of Cincinnati, accessed January 7, 2025, https://www.mpofcinci.com/blog/traceability-in-manufacturing-guide/
27. The Importance of Traceability in Manufacturing: An Overview - Insight Quality Services, accessed January 7, 2025, https://insight-quality.com/importance-of-traceability-in-manufacturing/
28. Complete Guide to Product Traceability - Blue Bite, accessed January 7, 2025, https://www.bluebite.com/circularity/complete-guide-to-traceability
29. Manufacturing Verification & Validation - Product Resources, accessed January 7, 2025, https://prodres.com/manufacturing-guide/manufacturing-process-design/verification-validation/
30. AI Safety: Principles, Challenges, and Global Action - Kolena, accessed January 7, 2025, https://www.kolena.com/guides/ai-safety-principles-challenges-and-global-action/
31. AI accountability | Carnegie Council for Ethics in International Affairs, accessed January 7, 2025, https://www.carnegiecouncil.org/explore-engage/key-terms/ai-accountability
32. Understanding AI Safety: Principles, Frameworks, and Best Practices - Tigera, accessed January 7, 2025, https://www.tigera.io/learn/guides/llm-security/ai-safety/
33. AI Risk Management Framework | NIST, accessed January 7, 2025, https://www.nist.gov/itl/ai-risk-management-framework
34. Ethical Application of Artificial Intelligence Framework - ACT-IAC, accessed January 7, 2025, https://www.actiac.org/system/files/Ethical%20Application%20of%20AI%20Framework_0.pdf
35. Traceability for Trustworthy AI: A Review of Models and Tools - MDPI, accessed January 7, 2025, https://www.mdpi.com/2504-2289/5/2/20
36. What is AI traceability? Benefits, tools & best practices - Data.world, accessed January 7, 2025, https://data.world/blog/what-is-ai-traceability-benefits-tools-best-practices/